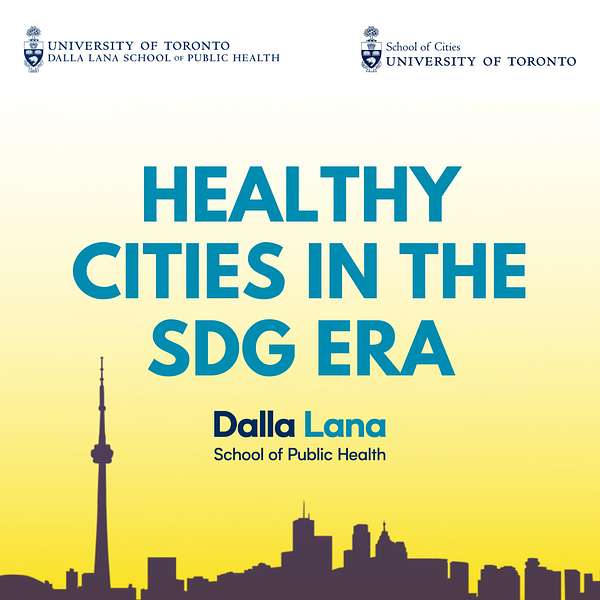
Healthy Cities in the SDG Era
Healthy Cities in the SDG Era
14. Industry, Innovation and Infrastructure
Sustainable Development Goal : 9 Industry, Innovation and Infrastructure focuses on building resilient infrastructure, promoting inclusive and sustainable industrialization and fostering innovation.
Dan Breznitz, is a University Professor and Munk Chair of Innovation Studies, in the Munk School of Global Affairs & Public Policy with a cross-appointment in the Department of Political Science of the University of Toronto, where he is also the Co-Director of the Innovation Policy Lab. In addition, he is a Fellow of the Canadian Institute for Advanced Research where he co-founded and co-directs the program on Innovation, Equity and the Future of Prosperity. Professor Breznitz is known worldwide as an expert on rapid-innovation-based industries and their globalization, as well as for his pioneering research on the distributional impact of innovation policies. He has been a member of several boards, as well as serving an advisor on science, technology, and innovation policies to multinational corporations, governments, and international organizations.
Vinyas Harish is a fifth year MD/PhD Candidate at the Temerty Faculty of Medicine and Dalla Lana School of Public Health at the University of Toronto. He is also a Postgraduate Affiliate at the Vector Institute and a Graduate Fellow at the Schwartz Reisman Institute for Technology and Society. He holds a CIHR Frederick Banting and Charles Best Canada Graduate Scholarship (Doctoral) Award to investigate how digital technology can support public health emergency response and promote resilient health systems. His research areas include machine learning, emergency preparedness, clinical and population decision support systems, and the governance of artificial intelligence in health.
CREDITS: This podcast is co-hosted by Dr. Erica Di Ruggiero, Director of the Centre for Global Health, and Ophelia Michaelides, Manager of the Centre for Global Health, at the DLSPH, U of T, and produced by Elizabeth Loftus. Audio editing is by Sylvia Lorico. Music is produced by Julien Fortier and Patrick May. It is made with the support of the School of Cities at U of T.
Ophelia Michaelides [00:00:07] I'm Ophelia Michaelides and this is healthy cities in the SDG era, a podcast about the Sustainable Development Goals and how research conducted by faculty and students at the University of Toronto is helping to achieve them. We're recording from Toronto or Tkaronto, which for thousands of years has been the traditional land of the Huron Wendat, the Seneca and the Mississauga's of the Credit. Today, this meeting place is still the home to many indigenous people from across Turtle Island and we are grateful to have the opportunity to work on this land. In this episode will look into SDG nine, which focuses on building resilient infrastructure, promoting inclusive and sustainable industrialization, and fostering innovation. There are several targets for measuring progress on SDG nine. These targets encompass enhancing scientific research and technological capacities of industrial sectors in all countries, supporting domestic technology, development, research and innovation, encouraging innovation and increasing the number of research and development workers. Innovation and technological processes may play an important role in the development of sustainable solutions to the challenges our world faces, such as pandemics and climate change. However, according to the United Nations, global investment in research and development as a proportion of GDP increased only 1.5% in 2000 to 1.7% in 2015 and remained unchanged in 2017. In Canada, R&D expenditure was reported to be at 1.5% in 2019, according to the World Bank. And spending is expected to stay at these levels. Thus, there remains a need for countries like Canada to continue investing in R&D that not only helps find solutions to the world's challenges, but also generates employment and income. At the same time, it is important to recognize that innovation should be equitable, benefiting all communities rather than the select few and populations. In this episode, we'll speak with Danny. It's about equitable innovation policy, especially among disadvantaged groups in particular. We will examine Canada's innovation landscape in the health care field and what can be done to improve our ability to innovate in an efficient and equitable way. Then we will speak with Vinyas Harish about a specific innovation in the field A.I. or artificial intelligence and its role in infectious disease and emergency preparedness. We will discuss the implications an increasing reliance on A.I. in industry partnerships and discuss the value of private public partnerships for innovation in global health.
Ophelia Michaelides [00:02:58] Danny Breznitz is a university professor and Munk Chair of Innovation Studies in the Munk School of Global Affairs and Public Policy. With a cross-appointment in the Department of Political Science at the University of Toronto, where he is also the co-director of the Innovation Policy Lab. In addition, he is a fellow of the Canadian Institute for Advanced Research, where he co-founded and co-directs the Program on Innovation, Equity and the Future of Prosperity. Professor Brezhnev is known worldwide as an expert on rapid innovation-based industries and their globalization, as well as for his pioneering research in the distributional impact of innovation policies. He has been a member of several boards, as well as serving an adviser on science, technology and innovation policies to multinational corporations, governments and international organizations. Welcome to the program, Dan. Thank you so much for being with us today. Please tell us a little bit about yourself and your research.
Dan Breznitz [00:03:56] So I'm Dan or Danny, because if you call me Dan, I probably wouldn't understand. You're calling me, Brezntiz. I'm a university professors and the Munk Chair of Innovation Studies in the Munk School of Global Affairs and Public Policy, where I also co-direct the Innovation Policy Lab at the University of Toronto. I'm also the co-director of Fellow of CFOR, the Canadian Institute for Advanced Research, where I co-direct the program on the Global Network and Program on Innovation, Equity and Prosperity. Right now, I'm also on secondment to our federal government as a Clifford Clark economist at the Department of Finance, and that's with the official titles. I think I now make sure I'm not insulting anyone who gives the money...On terms of research. Very simplistically, I'm looking at two things one about innovation policy. And how that trend can how can we make it more effective and effective, meaning a producing more innovation and also economic efficiency. But on the other hand, as you might have understood by the name of a problem, equity and prosperity, I'm extremely interested in trying to figure out. And that's why I said innovation policy and not innovation, how we can effect innovation policy in the way that there will be more, both economic efficient in terms of profit growth, but also the distribution outcome will be much more equal, especially with disadvantaged groups, the groups that are weaker in each society. And I'm looking at that both in terms of the let's call it geography locale, right? What different places can do. I am looking at the global system as a whole and therefore that what that means for specific societies and done I don't know, three, four books by now, god knows how many articles and my last book is an Innovation in Real Places. And the reason I mention it because the Writers Guild of Canada just gave it the inaugural Balsillie Award for Public Policy. And again, I don't want I want to make sure that everybody who's helping my research, especially research on equity, which does not get enough attention, get mentioned.
Ophelia Michaelides [00:06:44] Mm hmm. Absolutely. Congratulations. Well earned Danny. In listening to your bio and your descriptions I hear the word innovation a lot, so I'm wondering our listeners, as well as myself, might be curious to know more about what you mean by innovation. So what is your definition of innovation?
Dan Breznitz [00:07:07] So I think that's a great question and it's a great way to start this. And I think and the reason is that I think we have a horrible confusion in the world with, especially in Canada, about what is innovation and what is not innovation. So innovation is not invention. And if you and I will go back to a lab. Even if we find a molecular that actually works against the disease. And even if we sort of prototype it to show that and maybe even do a first clinical trial to show whether it actually works, that's still that's an invention. Innovation is taking those ideas and making them into reality. And not only in the novel, like bringing a new molecule up to the market, but all the way through from making it better, better figure out how to produce it, figure out how to distribute it, figure out how to service the people that get it. In all industries or from health to shoe make. And it is important specifically because of fact that it's continuous and it happens in the market. So I'll give you two examples. One is the coronavirus. It's lovely that we came up with a new molecular using a completely new technology called MRNA. But we now know that if we really want to control Corona, live with Corona, we will have to up our game. It's not enough for coming up with this. First of all, molecular, we have to figure out and we have in the last 18 months how to produce and RNA vaccines to the tune of billions, how to create new vials in glass and materials to put those vaccines in, because we necessary to rethink how to distribute that. And now we need to figure out how to make improved vaccines probably every 6 to 12 months and then produce them in the billions and then make sure that every human on earth has access to them. If you want COVID to basically become, you know, how we deal with the flu. So you could see that the real impact is not just that somebody came with a molecular, but everything that happened later. Okay? It's not that it's not important to come with invention, but if you want to have an impact in the real world, you need to innovate and you need to innovate all the way through. And it must be continuous. The second is what we are talking about, talking with now or using or Zoom ten or 12 years ago. We still had in the university special rooms to do, you know, telecommunication and special classes in which you had to have special cameras and special connections. I'm not talking about 15 years ago, just ten years ago, maybe even less. I remember I had to present in Korea and there was a special room on the third floor with a special camera, and it was really expensive. Now, all of us have Zoom and it's not new technology. This technology is 40, 50 years old. You just the fact that we have improved the technology, we improved cameras, we improved microphone, we improved data communication, we improved handling of memory to such a degree that now you and I can do that and we don't even think about the cost. And that's when it really has impact on society. Not, you know, when some somebody comes with a new gadget or not even when the gadget is being sold to only a few rich, trendy people and somebody become a billionaire, the impact on society happens when innovation is really widespread and we keep forgetting it.
Ophelia Michaelides [00:11:19] I, I had no idea that the, the innovation ecosystem was it was so complex much beyond just the the invention stage. That to me is very fascinating. And obviously it has very real impacts on, like you said, our day to day the life we've been living for the last two years under, you know, COVID 19 pandemic, our use of technology. Yeah, absolutely. Danny, I want to pick up on something you said at the very beginning around equity and the use of innovation for equity seeking groups. So in your most recent book, you argue for sustainable innovation based development and growth that communities can benefit from. So how can communities succeed at innovation, especially in reference to health and or health care? I'd be interested to know.
Dan Breznitz [00:12:18] So it's it's good that you mentioned COVID and it's good that you talked about the ecosystem, because the one thing that has changed over the last 20, 30 years may be 40, but most people realize it only when COVID happened and it certainly stopped is that we have moved to a completely new global system of production and with production innovation. So I know you really wanted a new car and now for about a year and you already paid for it but you know the Maserati didn't arrive because there's no silicon chips. And the silicon chips, we don't have them here because through that globalization, we only now figure out that there is only one country and only about two companies from that country that actually can innovate in the production, not on the coming of new designs, but in the production of semiconductor chips. And I'm talking about Taiwan and TSMC and UMC. Reason I mentioning it, but what really happened in the last 30 to 40 years that we have taken production of both goods and services, basically broken two discrete stages. So coming up with the end of let's let's talk about semiconductors or if you want to MRNA vaccines. There will be a company that will come up with a new more like a lot of a new design for semiconductors as soon as they finishes and this is basically a software code in both stages, they ship it over Internet automatically to somebody who actually know how to take that software code and make a real product out of it. But that product is not enough. Now, you maybe have a molecular and maybe you have the chip. It actually needs to go into a system of multiple chips. Just think about your smartphone, which is basically a supercomputer with 10,000 components or MRNA vaccines that have to be put in a lipid and be produced. And all of those then go and basically a way to think about it again simplistically, but it's a good way of thinking about it as if everything now has four stages the stages of novelity, the stages in which you figure out how to produce it, how to improve, how to design it into production, then how you improve in it and recombine it. And then the last stage in which you figure out how to assemble it all together. And if you look at the two industries we now talked about, advanced pharma and advanced semiconductors. Let me start with the semiconductors. We can look at Israel, the US. Please notice I don't mention Canada much Korea, Taiwan, China, maybe Singapore. All of them have extremely successful semiconductor industries. In all of them, you have almost all of them have the same companies working in them. And so you might think it's the same industry, but then you look and what you see is that those companies do extremely different things in those industries. So, for example, since we are hitting on semiconductors over time in Israel and Silicon Valley, they come up with new ideas to put on silicon. We now know that only TSMC can actually make it happen in Taiwan. In Samsung, they control niches. So anything that you want with memory or the control of your beloved touch screen. And then in China, they actually figure out how to take those 10,000 of components, constantly changing materials from glass to metal and coming up with a product that you actually will want to buy in the price you can buy and then ship it all over. And the same thing, by the way, happened with vaccines. People talk about Moderna are producing. It's not what Moderna that's actually producing the vaccines, it's Swiss. In this case, it wouldn't be Korea, it would be Switzerland. And we can go on and on. The reason I mentioned that it is that it tells you that you need to excel in innovation in different stages. Right, in order to be able to compete on those stages. What it also tells you, if you look at those stages is each one of them have completely these two different distributional outcomes. If you go to extreme Silicon Valley, to Israel and Silicon Valley itself, the only people you will employ are very high end R&D engineers. So the graduate of best universities in STEM so not people you should actually worried about. They will get unbelievable salaries and the lottery tickets called, you know, stock options. They, their financiers and maybe a few celebrity chefs. We'll be even million millionaires with a chance of becoming a billionaires. But then there's the other 85% of the population, and they don't even get reasonable jobs. In the book. I go into the details. We can talk about it later. It's okay if that's your decision as a society. Right. It's it's a I mean, I don't think I would argue that it's extremely difficult to even try to imitate Silicon Valley right now. But if that's you a decision, you should be aware of it. If you're successful, you're going to have massive inequality and figuring out how to deal with it beforehand, not when you know the proverbial shit hit the fan, so to speak, in different stages. Right. And focusing on those kinds of innovation, you decide also on who is going to be involved and how you're going to reimburse them. Wages, stock options. Right. So those are decisions about how you society looks like. And it's no surprise that Israel became one of the most innovative country in the world from being completely uninnovative but also became the second most egalitarian in the OECD to the second most unequal society among rich countries. And Taiwan actually stayed more or less equal. And Finland, by the way, if anyone wants another example of highly innovative country, will be king, highly innovative and still egalitarian Finland, which actually looks a lot similar to Canada, apart from the fact they know how to innovate and we don't.
Ophelia Michaelides [00:19:14] Mm hmm. Maybe a different stage, right? Maybe he look into our future. Who knows? But I'm fascinated by the by the link between equity and innovation that you've described and some of the fascinating exemplars that you've given. It was not lost on me that Canada was not in the list there. In several of the examples that you gave, I'm curious to know what the current health innovation landscape looks like in Canada as it stands, and what can be done to make health innovation perhaps better in the future here in Canada.
Dan Breznitz [00:19:58] So Canada is not as a country even in the game.
Ophelia Michaelides [00:20:04] Mm hmm.
[00:20:06] So let me just and we can talk about examples in different industries, in different stages. And in the book, I have, you know, industry in Italy, bicycle making and everything, high tech. Right, in different parts of the world. Canada as a country. Right. We might be able to talk about specific places is not really in the game of innovation, which is actually surprising because in the last 30 to 40 years, we have became the most highly educated country in the world. Some of my colleagues in economics would argue with, I'm counting colleges here in not just universities. And my answer to them would be that it's just like R&D intensity statistics. If you're number five and number one, it doesn't really matter, especially if you've been number 1 to 5 for ten years, you're at the top. The same is for our research, and that's really important. By the way, for health. We have created a public research infrastructure between universities and other organizations, which is as good as it gets, actually punch way above its weight. If you look at invention that became really important innovation, especially in Health Canada, where we completely fail is what we do with those highly educated people. And those ideas are. For example, one of the most common statistics is to look at the to fix one investment of a private sector in R&D and innovation, you know, ratio for GDP. Canada is the only the only we see the country that has declined year by year for the last 20 years. And I'm very happy to say we now get really officially the wooden spoon, because as of this year, we are now below Poland. Okay in that intensity. So our private business, we have a classic market failure in the private business, but unfortunately not enough people talk about because we constantly talk about our higher education research, blaming the university, blaming private business, not does not invest in R&D or worse of a private business, does not even invest in the technologies and the private business. And you can see it also in the median wage. Canadian median wage for the last 46 years is stagnant because the business model of most Canadian companies now is to take a highly educated people, pay them below market price, because we know what price that we get as wages and put them to work in a minimally technologically advanced capital, but sort of because we are so overqualified to have enough productivity that the profits for those companies keep on going. So we have a disastrous market failure, which we don't even talk about. We constantly talk about our innovation failures, but without fixing the private market, it's game over for Canada. And until and it was so slow and we haven't noticed, but now it explodes that whatever we are going to do something about it. I don't know. And then comes health. So the problem and we have several problems with health. One is health now, isn't IP on the top end is. There's a health on the top end and there's health in which you can actually have a sustained creation of very good jobs. And they're not exactly the same. Remember Moderna? and then I said, somebody produced for me Swiss and other companies. So on the high end, it's a complete IP game, intellectual property. We have been losing this game. We actually probably we haven't been playing this game for about 40 years, and especially since a few trade agreements like NAFTA, it's have gotten worse. So my colleague Dan Traxler and a few others, just look at the royalties on IP trade balance between just the U.S. and Canada since NAFTA. And it moved from us being on the top to us being on the bottom to the tune of about 5 billion. And then if you look at the overall trade of Canada, there is another almost two billions of bits of IP with Canadian companies and individual pay to use. The sad fact is with a lot of our IP in health and other places like artificial intelligence is actually based on Canadian inventions. Okay. In health, it's even more on that level. It's even more complex because we don't really no longer have companies that can play this game. Our health system, while public, is actually fragmented because it's controlled by the provinces and with some federal. And it's also fragmented because there is a grunting council of hospitals as. There are some interesting experiments, especially since some people have figure out whether we cannot win the IP game. So we might want to play open IP. So with the experiment happening around the University of Toronto, experiment happening around McGill, but there were not big enough. And I'm also not quite so sure that this is the game we can win. We need to be a lot smarter, right? I have looked at the size of NIH and I've looked at the size of the Canadian federal budget. And this is not a game we can win. Period. And remember, I just said the NIH, I did not say anything else in the US. So what is a game? And by the way, even if you win, what kind of jobs will create? And that's, I think, where our failure is. We constantly underestimate what I'll call here a Taiwan model. Taiwan and TSMC and UMC and other companies have created magnificent jobs and wealth for a country highly distributed by saying, we are not going to fight this game. But every time, let's say an MRNA and a medicine, because this is going to transform medicine, not just vaccines, comes with people who know actually how to produce the product of Taiwanese companies. And we instead of figuring out that this is a new game to have in town, focus almost obsessively on creating facilities for one vaccines for one company. So Pfizer will have a vaccine facility here. Well, then, no, we need to create the capabilities in which whenever I call it contract manufacturing, we need a Canadian MRNA and a TSMC. So I don't know how you want to call it Canadian MRNA... M production facility. Whatever. Whenever somebody. Anywhere come off a new MRNA molecular and try to figure out how to produce it. They come to us. And the same can go for almost everything we can talk about. A very interesting model actually does not happen in Toronto. It happens in Hamilton around McMaster University, who also, by the way, if you look at their history, have innovated in the way we now teach medicine and problem solving and have also innovated in the way in which we try to innovate in actual medicine, cold medicine, evidence based medicine. Both of them are from McMaster. Both of them means a different thing about how you treat and how you produce treatment, which is much more, let's call it line workers, meaning the practitioners. And not just, you know, there's an R&D facility with scientists, they do other things. And, you know, if a medical doctor just say, get this pill and put it into people, but it's much more coming through the practitioners and use and you can see the companies that come from or have been coming through McMasters, they are creating platforms for all kinds of multiple cancer medicine. We might sometimes have to come up with molecular themselves or somebody will give them money. But you look at the real inventions. That's where every platform companies I think it's a very interesting experiment for all of Canada and not just in medicine. I think we are destroying it at the moment because we have now involved so much venture capital into it and so much of that. Then to capital comes from the US and the only game with the US venture capital are interested in playing is the game in which Canada is not going to win. So how do we take the things we can already do? McMaster is one example. CMO is contract manufacturing in health are another open science medicine, right counter maneuvering, the patents and IPR. How do we scale those up without falling into the trap when somebody offers a huge amount of money and transform us to an outpost of Silicon Valley? That's an interesting question.
Ophelia Michaelides [00:30:39] Mm hmm. I find that fascinating. And and your last example, you know what? What is unique about the McMaster, this this Canadian, you know, this process. And and how can that be? How can that be leveraged? Right. To ensure that innovators can successfully grow and prosper here in Canada?
Dan Breznitz [00:31:03] Let let me let me say something. I think about McMaster and why that happened in McMaster. McMaster is very new to the game of medical school and medical research. It had this massive monster called University of Toronto and the hospitals there and the right nearby. And they knew that a very new sovereign were not wedded to the mainstream and b) they new and they had the leaders who understand that, that in order to be as good as they can, they have to to do things differently. And for now at least, fair kept it right. You will see over studies around demographics and and data and population data. And Canada needs to stop thinking. And I've been in the US for many years, my Ph.D.s from M.I.T., I taught in Georgia Tech. I, I've been in Stanford and Barrett. We are not the U.S. we will never be the US. There is no chance of us ever winning. If we try to compete head to head with the U.S., we want to create jobs and a healthy health and industry and healthy society. And in terms of health, not just, you know, we need to figure out what is a Canadian model. And because we are also very diverse and it's province by province, we can run multiple experiments, but instead. We we just look at that at the US and say, oh, you a master, we'll just follow you. Thank you, Yoda, for telling us how to become Jedis. And by the way, for taking away our money, our invention, and our best scientists.
Ophelia Michaelides [00:32:58] Right. Right. So in listening to this, how can we here create an in an enabling environment for for all these things that you've described? And, you know, often, you know, when I think enabling environment, I think policy, the policy sector, you know, you mentioned, you know, your work in innovation policy at the very beginning of our of our talk. So how can policies, whether they be, you know, focused on health, science, innovation itself, how can they be designed to spur good innovation in the field.
Dan Breznitz [00:33:36] Is one, we are really good in invention and I don't think we should stop being good. Didn't mention I mean very clear that we need one easy thing that we need to do is to figure out a better way of how those inventions, you know, from the lipids that you now have to use in order for that many vaccines to new vaccines, to new medicines, how do they translate into products? Real fing inventions in color. That's one up. In order to do that, we have to figure about new financial instruments, but we also have to figure out how to deal with IP intellectual property. And there is no way in hell that we can win this game if we are not becoming much more creative. And if we if if we do not figure out, you know, we are in a strategic disadvantage, how do we work with it? And we, you know, from patent collectives to open science to various thinking about prior research to attack back were companies that will attack creating a space for Canadian inventors to become innovators in Canada. Okay. The second is stop being obsessed about the new molecular. I talked about Taiwan. Forget Taiwan for a moment. Look at Switzerland. Look at Denmark. Even look at Ireland. Look at the American biotech industry. And then calculate how many great jobs and billions of dollars American biotech companies or industry actually create. Not in India and China, but in Switzerland, Denmark and Ireland. Countries where. You know, we can compete with them easily and then after game and saying, okay, those are in the global industry, right? Health industry was a position of power. You know, intel, CPU, giant bicycle. You know, framework of is now that in those industries they will have to figure this out. And they're not cool. They might be boring, but they need a huge amount of innovation and different financial institution than, you know, Silicon Valley and Wall Street venture capital. Let's figure out what companies we have that can play this game. Figure out what laws and regulation they need in order to grow in Canada and what kind of other things like finance they need and make sure that they can do that because they'll produce tens of thousands of jobs for Canadians who are not only going to have, you know, M.D. from our best in the first place.
Ophelia Michaelides [00:36:59] Right? Yeah. Well, it sounds like there's there's tons of potential for policy makers to play a coordinating role, a supporting role in neighboring enabling role in all of the things that you just mentioned. Danny, I want to thank you for giving us a tour of the landscape, the innovation landscape here in Canada and abroad, and to really show us what is possible for future generations of Canadians. And I believe that, you know, with all of these these ideas in the pipeline and hopefully our get much closer to implementation so that we can we can prosper as a country and certainly, you know, mitigate effects of future crises, which is something that we've seen over the past two years. So, Danny, I want to thank you for joining our episode. It's been such a pleasure.
Dan Breznitz [00:37:53] Thank you so much for having me. And I have a lot of optimism for Canada, but we need to we need to start thinking like Canadian instead of northern Americans.
Ophelia Michaelides [00:38:06] Yes. Words of wisdom. Absolutely. Thank you.
Dan Breznitz [00:38:11] You're welcome.
Ophelia Michaelides [00:38:21] Vinyas Harish is a fifth year M.D. Ph.D. candidate at the Tammany Faculty of Medicine at the Dalla Lana School of Public Health at the University of Toronto. He's also a postgraduate affiliate at the Vector Institute and a graduate fellow at the Schwartz Reisman Institute for Technology and Society. He holds a CIA chair, Frederick Banting and Charles Best Canada Graduate Scholarship Doctoral Award. To investigate how digital technology can support public health emergency response and promote resilient health systems. His research areas include machine learning, emergency preparedness, clinical and population decision support systems, and the governance of artificial intelligence in health. Vinyas, welcome and thank you for taking the time to be on our podcast today.
Vinyas Harish [00:39:06] Thanks so much for having me.
Ophelia Michaelides [00:39:08] Vinyas, we'd love to know more about you and your research.
Vinyas Harish [00:39:13] Sure thing. So I'm a fifth year M.D., Ph.D. candidate here at the University of Toronto. And what that means is it's a lot of school, but I've done the first two years of medical school, and those are the preclinical years we'll see learning in the classroom. And then I transitioned into my Ph.D. in clinical epidemiology at the Institute of Health Policy Management and Evaluation at the Dalla Lana School of Public Health. And. You know, before I tell you a little bit about my thesis, before I started this program, I did my undergrad in computer science with the emphasis on biomedical computing. So it's kind of like biomedical engineering. I took courses across the life sciences as well as, of course, computer science, so things like machine learning, software development as well as on the other side. Global health, organic chemistry, the whole range of things. And that's when I first started getting interested in this intersection between technology and medicine and health care and health systems. And when I got into med school, I was interested about how emerging technologies such as artificial intelligence could be used to help us understand and respond to emerging infectious diseases. So I say that I was interested in this area before it was cool and relevant. Yeah, I could have never predicted the global pandemic to happen in my Ph.D. but here we are. And so in response to that, I sort of pivoted the direction of my thesis because I was early enough in my graduate training to really look at how health systems can use digital technology. With a focus on novel analytic methods like artificial intelligence, machine learning to respond to crises. And I want to look at that in a couple of different ways. So I'll speak more about this in detail later in the podcast, but about how we can make partnerships work better between the private sector, private technology companies and health organizations, as well as do some work developing algorithms myself and actually working with Data Holdings, both at the Institute for Clinical Evaluative Sciences, Health Administrative Data, as well as some cell phone based mobility data to answer questions related to the pandemic and ultimately try to move the needle on how health systems can. You know, leverage the data and the novel technologies they have at their disposal to respond to these major crises.
Ophelia Michaelides [00:42:12] Mm hmm. I mean, is your your work is so fascinating and certainly so so relevant for the world that we live in today at the present moment. And as you describe, your work is very much at the intersection of many fields of knowledge. I was wondering if you could explain to us briefly how artificial intelligence or A.I. is used in the field of infectious diseases and emergency preparedness.
Vinyas Harish [00:42:41] For sure. So I'll start by just providing a brief definition of artificial intelligence and machine learning. Because I'm not sure if your your listeners are too familiar with it. So artificial intelligence is a very broad area of research spanning now everything from psychology to neuroscience to computer science. But really, a sort of formal definition I often go back to is it's the design of intelligent agents that receive precepts from their environment and take action. And I think a lot of times when people hear the term artificial intelligence, they sometimes think of Terminator style robots. Yeah, but that's what we call artificial general intelligence, which is pretty much relegated to science fiction at the moment. But what there are real world examples of are what we call artificial narrow intelligence, or these sort of intelligent agents that can do a small subset of tasks really, really well. For example, I mean, DeepMind recently, last week, I think came up with an algorithm that can control a nuclear fusion reactor. Other algorithms play board games like the game of go or chess. But just because an algorithm can play chess doesn't mean it can control nuclear reactor and its performance doesn't generalize across tasks like. We think with these sort of science fiction robots and within A.I., there's a sub area called machine learning. And machine learning really blew up. In the in the 2000s, especially around 2012, thanks to another sub area called Deep Learning. So you could really imagine these as you know, those Russian nesting dolls where you have one thing inside of another and so is the broadest one. And then you have machine learning and then you have deep learning as a subfield of machine learning. And machine learning is all about developing algorithms that improve with experience. And so you generally provide these algorithms with a bunch of data, a mathematical approach to learning from the data and. Eventually they get better at a task based off of the the data that you provide. So now that these definitions are out of the way and I'll talk about how I could be used an infectious disease emergency response and refereeing this concept that I really like called the the Gartner analytic value escalator and I think it's in the show notes the link to the image but I'll I'll briefly describe it. So on the x axis you can conceptualize this notion of difficulty. And on the Y axis, you can imagine an axis called value. And so as you move up into the right tasks become more valuable, but they become more difficult as well. And so along this this escalator, we can have four stages of increasingly difficult. Tasks, but also more valuable to us for a business or, you know, in our case, for a health system. So at the very bottom, there's descriptive analytics. What happened then? You have diagnostic analytics. Why did it happen? Predictive analytics. What will happen? And prescriptive analytics. How can we make it happen? So artificial intelligence has examples pretty much along this entire spectrum. So at the ground for descriptive analytics, what happened? This is when we think about something like COVID, we think about examples in infectious disease surveillance, picking up cases, keeping track of done. And there's a Canadian company called Blue Dot, actually based in Toronto that received international attention because their algorithms detected the first cases of COVID. At the time what was an unknown pneumonia from the Wuhan wet market that has now of course evolved into the COVID 19 pandemic. And so this company has algorithms and a type of machine learning algorithm that falls under the family of natural language processing. And so these algorithms analyze news articles from all over the world pretty much 24/7 And they detected this news article in the Chinese media speaking about this. Novel Pneumonia of an unknown origin. And so that's descriptive analytics that give you an example there. Diagnostic analytics. Honestly, I don't know if there's to too much here in this space. I often think this sort of diagnostic analytics, why did it happen is is very much the role of traditional epidemiology, traditional statistics. Is there an association between X and Y or maybe in, you know, very mechanistic studies of why something might be occurring? So what kind of skip the diagnostic analytics? There. But in terms of predictive analytics, of course, a natural example could be forecasting, right? So forecasting cases, case counts in a in a certain jurisdiction. And forecasting is just a very common example of a machine learning problem. And finally, with prescriptive analytics, there's another group of machine learning algorithms that fall under this window of reinforcement learning. So these algorithms are really cool because you could place this algorithm in a environment and it needs to learn essentially how to make decisions. So one really interesting example that came out during the pandemic was an algorithm to allocate ventilators across counties in the United States based off of predictions of case burdens. But also, okay, if you predict this many cases and we expect them this many people to go to an ICU, we should send this many ventilators to this hospital in New York City versus this many to another community hospital in upstate New York. And then based off of those outputs, here's what might happen. And then later on, we should move these ventilators somewhere else. So this algorithm in this case responds to a dynamically changing environment to actually help make decisions. And as you can imagine, that's extremely valuable but very difficult to do. Right. So that's a, you know, overview of some examples of how artificial intelligence can be used across different aspects of emergency response when you're talking about something like a pandemic.
Ophelia Michaelides [00:50:06] Mm hmm. I mean, there's a really appreciate what you just explained, and particularly the nuance of what we mean by a I think, you know, the general public, we we throw around this word, perhaps not always understanding the nuance of the subcategories. And this is a very vast field with so many applications. And I really appreciate how you explained how it can be used for specific purposes and the exemplars of each that are very relevant to perhaps the data and the evidence. And then the predictions that we hear, we read about ongoing with respect to COVID 19. And so it's very helpful to hear the the the tools that are used to generate this information. And one thing I wanted to pick up on is often in the literature discussions around AI and health care specifically mention ethical A.I.. And so that being a AI designed to mitigate bias and ensure the benefits of eight AI are shared widely. So when we're talking about ethical A.I., what is that? And how do we ensure that AI is ethical, in fact?
Vinyas Harish [00:51:27] Yeah, it's a it's a big question. And now I think it is turning into an entire field of study in itself. But I can kind of break it down into ethical AI should say there's a conference in this area called FACCT or Fact, and I break down ethical AI into the three composite letters here. So the F stands for fairness, a, c, c stands for accountability, and the T stands for transparency. So the first category is fairness. And even within fairness, there's a lot of different ways to define fairness. And they can get really nuanced mathematically because to a mathematician, fairness may mean something to a legal scholar, fairness may mean something else. But especially in the health domain, I think a grounding way to sort of conceptualize fairness is around this concept of health equity and something I'm sure your listeners know about are the social determinants of health and the fact that certain marginalized groups have worse outcomes and worse access to care based on these social determinants. And so when we develop machine learning models and in our own research, one way of looking at fairness is, let's say you have an algorithm to predict the risk of a person developing diabetes in five years. And so there's algorithms developed from I mentioned ICS earlier that can ICS is an organization in Ontario that holds health administrative data. Every time Ontarians interact with the health care system, they they generate data in these databases held in ICS. Yes. And. One way to look at if a model is fair is for patients who may be at different ages in their forties versus their fifties versus their sixties. If the model can predict the actual risk of them developing these diabetes complications ah [...] Evenly based on these age categories, right. So one age does not have much worse performance than another. Or let's say in Ontario, we don't do a good job of collecting data on race or ethnicity. It's a whole other conversation in of itself, but especially in the United States, you can imagine, okay, if you have an algorithm to predict the risk of diabetes complications for black patients versus white patients, you do not want to see a big performance gap in your algorithm for black patients than for white patients, or frankly, vice versa for for whatever reason. And there are ways to to explore that and try to make your model more fair. I'll link to an excellent paper that was published in Science a couple of years ago that sort of broke ground on this area and realized that health insurance algorithm used in the United States was it had this exact problem. It did not [...] Perform equally in black patients, as it did white patients, and actually prevented them from getting the care resources that they actually needed. So I'd recommend a listener who is interested in that to check the show notes. And take a look at that paper. And so that was the F for fairness. The next is accountability and accountability, I think of in a lot of different ways. You know, even at one level, it's who's actually at the table, who's coming up with these algorithms. I think Silicon Valley traditionally has gotten a lot of flak for, you know, being a group of. CIS heterosexual white men that created these technologies that the rest of the world would be using. And there's a big moment now to diversify people and technologies, as well as lots of other sectors. To get to the fact that, you know, people have different lived experiences and the lived experiences they bring to the table eventually work their way down into the algorithms that they develop and the decisions that are made. So one way of remaining accountable. Is also, you know, just fundamentally who are the people who are making these algorithms that are now being used all over the world? And another dimension to to look at could be workflows. So especially in the context of something like health care. Does it make sense for an algorithm to be automatically making treatment decisions, or should there be a physician or some other clinician in the loop at some point? And if something goes wrong, who's who's liable for that? I think it's an area of increase in work, and that could be a dimension of accountability as well. And finally, transparency. Oftentimes people break it up into if AI is explainable, if we can understand what's going on under the hood. A common criticism with modern methods, especially deep learning, is that they're called black boxes. It can be difficult to understand how you're getting the prior predictions that you're actually getting. And now if you're using these algorithms for. You know, mission critical systems like controlling a nuclear reactor or allocating ventilators. It might be problematic if you actually don't know what's going on under the hood. There's a lot of again, I think I'm going to be saying this throughout the entire podcast. There's an entire field dedicated to this and a lot of debate around it. But thinking about the role of potentially explainability, I think is one aspect of transparency. And the last thing that I'll mention, especially in the health sciences, is thanks in part, largely in part to the movement of evidence based medicine. There's been a lot of rigor in the field about how to conduct research in terms of best practices for conducting different types of studies. And so there are going to be soon a set of guidelines released for machine learning in the health sciences to try and make it as rigorous as possible that came from. I think it was a through a Delphi process to actually establish consensus, to say, okay, researchers should do X, Y and Z to have the highest quality research possible. And so ethical AI is a huge field. I often break it down into fairness, accountability, transparency. Each of those are fields in themselves. But that's a brief overview.
Ophelia Michaelides [00:59:06] Mm hmm. That's so helpful. And I want to thank you for demonstrating the limitations, I guess, of technology and our responsibility to designer tools in a way that are reflective of our values. And, as you mentioned, these various dimensions that are important. And I'm glad to see that this is very much a work in progress. And these are burgeoning fields. I mean, yes, I wanted to now turn our attention to one of your thesis projects. As I understand, one of your thesis projects involves studying partnerships between health organizations and private technology companies during COVID 19. What have you learned from this project and what, in your opinion, makes for a successful partnership?
Vinyas Harish [00:59:55] Yeah. Thanks. I'm really glad to have this opportunity to conduct a qualitative study in my Ph.D. It was something that I wasn't really exposed to too much earlier in my training, but I thought it...It's one thing to actually be able to develop these technical algorithms, but it's another thing entirely to think about how we can actually get them into practice. And like we spoke about before, you have this trade off between value and difficulty with these different types of methods. And all along the way you should also try to think about how to do this ethically. And now you're doing all of this, which is difficult enough in normal times, but in the context of an emergency. And so through this study I interviewed with the help of an amazing, amazing undergrad research assistant Tong, just wanted to give him a big shout out here. We interviewed, I believe, 25 individuals across three different partnerships between private technology companies, two hospitals and a public health organization. And we found a bunch of interesting things about how they were actually able to do all of this to deploy technologies. They weren't necessarily artificial intelligence. They were they were different. But I think the lessons are largely transferable. How they navigated the barriers and enablers of responsible governance to deploying these tools in the context of a crisis. And, you know, unsurprisingly, the first thing that we found was a pandemic health emergency creates an immense pressure with time and resources and one participant akin to a big... COVID is just a big giant star that pulls every...every possible resource towards it. And so you have to make do with a lot less in terms of maybe the ability to to pilot certain features or to test things out or even in terms of the capacity people have to to work on a certain problem. And so given these immense time resource pressures. We found one of the most important things to do is to have a clear understanding on the core problem that needed to be solved. And if that isn't figured out, the whole thing goes out the window. You can't do much else beyond that because it really is the foundation for the entire partnership. And it's one thing to identify what the core problem is at the start, but in a rapidly changing environment like COVID. The organizations also needed to make sure that they remained aligned through the course of their partnership, and they had a set of governance structures and processes to help them do that. That gets a little too technical, a little too dry, but that was basically the core challenge that they needed to solve. And I think something else that was pretty surprising to me and to do this work, we we leaned on some theoretical frameworks for, for governing the deployment of novel technologies in the health sciences. And something that wasn't actually mentioned in the framework that we used was an emphasis on, I call it people skills, really. You know, it's like the basic things like empathy, like patients, because everybody has been dealing with burnout. Everybody has been dealing with the constant workload of COVID. And so. A partnership is only as strong as the people that it involves. And healthy, motivated teams are just so vital to actually carry this partnership through because without it, again, everything falls apart. And so that manifested in a lot of different ways. For example, leaders being really hands on, not just when things are going well, but when the partnership is actually facing problems. And they need to navigate a certain challenge. Leaders need to lead and step up to the plate and and help their teams navigate these problems. And, you know, in one sense, it all sounds very straightforward and obvious, but to be able to rigorously arrive at some of these findings and be able to characterize them and add some nuance, I think. I learned a lot. Found it very, very interesting. And I hope eventually the Journal reviewers and editors agree they find it valuable.
Ophelia Michaelides [01:05:12] Mm hmm. You raised some very important points, whether obvious or not, you know, just around problem identification and healthy relationships. You know, in what makes a successful partnership. I'm so glad that you were able to conduct this work with your team. I also want to mention that so much of this work also has a very direct impact on other SDG targets. I know today we're speaking of a very specific SDG nine. However, there is an SDG on partnerships really that focus on strengthening global partnerships for sustainable development. In fact, one of their targets explicitly mentions that the promotion of effective public private partnerships, which I think is your work, very much, gets to one of the components of what makes that work. Vinyas, looking ahead, I'm wondering, how do you think industry partnerships will impact the future of global health after or perhaps in the future vis a vis the COVID 19 pandemic?
Vinyas Harish [01:06:24] I think it's it's huge. These partnerships aren't going anywhere because when we think about these sorts of wicked problems, climate change, equitable development, misinformation, I mean, you name it, these are problems that are just too big for one sector alone, whether it is the private sector, whether it is. Non-governmental organizations, philanthropy, whether it's academia, you know, meaningfully moving the needle. Across all these different challenges that are really important for the SDGs will require. Collaboration among all these different partners because. They all bring different skills and abilities to the table. And I think the challenge or the opportunity here is to think about what we've learned from COVID, the biggest health emergency in arguably 100 years, and to start to really think about how we can make these partnerships as effective as possible and. Really? You know, like I said, move to move the needle and. On these big problems, especially, I think technology companies over the last 20 years have had a growing influence on our lives in a lot of ways. Good. In a lot of ways. Bad. But they're not going anywhere. That's the that's the take away. Right. And so I think. The work that needs to be done now is, I think, a lot more of what I started to do in my Ph.D. about thinking about how we can bring all of these collective resources to bear. And I spoke briefly about, you know, we're wrapping up the project now and we're hoping to submit this to academic journal, but that's only one dimension of knowledge translation.The more challenging one and the one I'm much more interested in the future is how do we actually get practitioners to learn from these and incorporate these into into what they do day in and day out. And I'm fortunate this work was supported by the Vector Institute, Schwartz Riesman Institute for Technology and Society, The Ethics of AI Lab and all of these supporters are interested about crossing the theory to practice gap. And I think that's what we need to do more of and think about research that can help us do that effectively, because I'm so glad to see Partnership for the Goals being in the SDGs, because I think you can't really have too much else without without that one.
Ophelia Michaelides [01:09:20] Exactly. Absolutely. As, as an enabler. And, and certainly the applications are endless from from from when I when what I've learned from what you've shared today, I mean, yes, your work is incredibly important and and complicated and complex. And so I want to thank you for for all of the work that you're doing and for sharing your research with us today.
Vinyas Harish [01:09:48] Yes. Thanks so much for having me. I really enjoyed speaking with you today.
Ophelia Michaelides [01:09:52] And likewise. Thank you so much for for sharing everything on today's show.
[01:10:03] The conversations with our guests today have shed light on the importance of inclusive and equitable innovation. As Danny explained, the American model of innovation also also called the Silicon Valley model, perpetuates inequality in society. Countries like Canada should move away from this model and seek inspiration from other, more equitable models of innovation, where such types of innovation can produce more jobs for average citizens rather than a specific elite in society. This episode also discussed the benefits of public private partnerships. As Vinyas noted, a collaboration between public and private actors as much needed as they can, pool resources and ideas that could provide solutions to some of the challenges the world is facing today. However, there are potential pitfalls to this approach, including a possible difference in approaches to managing such a partnership between two very fundamentally different partners. One such mitigation to this would require that partners across the theory to practice gap by learning to effectively integrate knowledge into real world practice. Healthy cities in the SDG era is made with the support of the School of Cities at the University of Toronto, whose mission is to bring urban focused researchers, educators, students, practitioners and the public together to explore and address complex urban challenges. We would love to hear your thoughts on healthy cities in the SDG era. If you enjoyed this episode, please rate, subscribe and share to help others find the series. You can find healthy cities in the SDG era on Spotify, Apple Podcasts and the Dalla Lana School of Public Health YouTube page, as well as our Center for Global Health website. Join us for our next episode where we'll look at SDG 16 Peace, Justice and Strong Institutions. Thank you for tuning in, and we look forward to speaking soon. Take care.